Interviews
A Chat with Frontiers Speaker, Sergei Kalinin
October 11, 2023
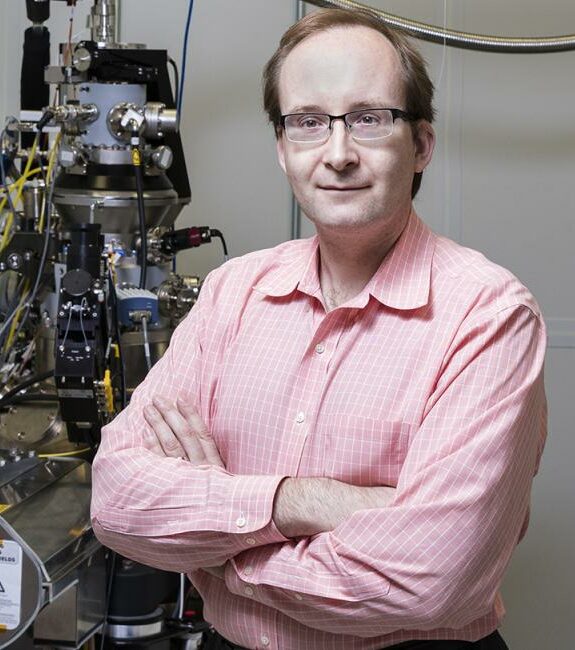
Frontiers in Nanotechnology speaker Sergei Kalinin gave us a preview of his upcoming seminar, how machine learning is already part of our everyday lives, and where he wants to take it next.
Q) The title of your upcoming lecture is “Bits to Atoms and Atoms to Bits: Autonomous Science and Atomic Fabrication in Probe Microscopies.” Did I get that right? Or did I butcher it?
SK) It’s a mouthful, yes.
Q) Machine learning is something people use in their daily lives without even realizing it. How would you put it in perspective?
SK) “Do you have a cell phone?” Let’s assume that the answer is ‘yes.’
“Can your cell phone do something useful?” The answer would probably be, ‘Yeah, I can do a lot of things. I can connect to my friends. I can watch TikTok. I can call Uber. There are so many things I can do using my cell phone as a way to communicate with other people.’
And then I’d tell them their cell phone is using machine learning. It improves an image, suggests a potential video on YouTube, or finds the Uber driver that you’re going to call. But the problem is that right now, it is literally you, the person who does all the work.
Q) So your goal is to have machine learning take on more of the work instead of making a recommendation?
SK) The way I see my science and my scientific interest, rather than cell phones telling humans what to do and humans doing the job, I want to create scientific systems where the machine learning agent can run the instrument directly and learn something about nature. We have a lot of tools in our everyday and scientific lives that allow us to perform actions. It can be a synthesis in the lab or it can be a microscope that allows us to observe and study things in detail. The problem is that it is still not machine learning that runs them. It is humans who actually operate them directly.
Q) What are the limitations of something like ChatGPT in the scientific community?
SK) ChatGPT and related technologies are exceptionally useful but have been trained on data provided by humans; they don’t get the information from somewhere fundamentally new. The measure of success of science is finding something new. I can say that ChatGPT will not be able to find anything new beyond the things that it will be trained on.
We always need some way to systematize what has been done before, help us do the simulations, and help us do the image analysis better. But science is fundamentally doing new things, and that’s something where machine learning, at least, in my opinion, is not going to be very helpful.
A) Do we have to worry about a machine uprising?
There is a joke about it; imagine that there is a robotic behavior rebellion. How would the robots fight this war? But you would easily say that when it comes to that, the vast majority of the previous wars have been won by the bow and arrow and swords. So they train from examples and find if the robots want to take over humanity, they should use bows, arrows, and swords because that’s how most of the previous battles were won.
Q) What is your approach to machine learning for the experimental sciences? When would you find it to be most useful?
SK) When we can introduce machine learning as a part of our everyday tasks. About half a year ago, I realized we are going from a microscope controlled by a human to a microscope that is controlled by a machine learning agent, that is controlled by a human. This is roughly the same transition as from riding a horse to driving a car. It’s not the transition from a Toyota to a Lamborghini because they have the roughly same engine with the same control principles. If you can drive one, you can pretty much drive another one. The difference between machine learning being a part of the experimental workflow and human running this workflow is the difference between the horse and the car, not between two different sorts of cars.
Q) Tell us about your journey to the University of Tennessee. You’ve had a couple of stops along the way, including a year abroad at Amazon.
SK) I got my first paper published when I was in high school. When I was an undergrad back in Moscow, active research was part of the equation from essentially year one. When I got a Master’s degree, I had about 20 to 25 publications under my belt.
I moved to the University of Pennsylvania, then I got a position in operations at Oak Ridge [National Laboratory] when one of the Department of Energy NSRC Centers was being developed, and it was the opportunity to build the scanning probe microscopy program. I was in the right at the right time.
Q) What was your goal when building that program?
SK) At that time, the dominant trend was to improve the spatial or functional resolution and make a microscope that can see the nanoworld better. Working with some of my collaborators, we decided that we wanted to take a commercial microscope and make it collect more data.
There are fundamental reasons why you can collect more data. There is a term called ‘oversampling,’ which means your microscope can collect more data than you know how to use. A typical example of over-sampling is we have things like stress spectrum modulation, which is how microwave communication works. For physical imaging tools, it is almost the same thing, so we can use them to collect much more information than the classical methods allow us if we run them a little bit differently.
We found a way to run our microscopes in a regime that gives us much more data than before. We can look at this date, and we see that it actually shows us something, but we don’t know what this means. The problem is that once we deal with the new measurement methods, there is no one to tell us what we are looking at or how to interpret it. In many cases, we answer this type of question by using physical models to translate the data into the language of physics that we understand. But we need a model for that. The biggest problem at that time was finding the algorithm that worked and making sure that you have a sufficiently powerful computer that allows you to run this algorithm.
Q) How did deep learning impact your research?
SK) I had some early papers when I trained the model by the neural network by theoretical model, and then I applied it for the analysis of experimental data. I’m happy that I wrote the paper about it, but the primary outcome was that it failed rather miserably. Now, we know that this is what is called epistemic uncertainty. If your model does not describe your data precisely, neural networks will likely fail. Physics-based methods allow you to jump over this type of gap, but neural networks cannot.
Then deep learning appeared, and that was a game changer. All of a sudden, we can actually use machine learning to solve the problems that traditionally took us a lot of time and effort, something as simple as finding the atoms and all the images of some object.
One of my postdocs realized the first Keras deep learning network in 2016, barely four years after deep learning appeared. And then, we compared the result of this deep learning analysis to the classical way to reprocess the data. After that, I basically focused my group on the application of machine learning for the experimental scenarios.
We started to do a lot of the deep-learning analysis for images. For the last five years, we have worked on the active experiment when the machine learning algorithm should tell the instrument what to do.
Q) What led to that decision to take a year off and work at Amazon?
SK) I needed to grow professionally. I knew that I needed to start to develop a normal university-based program. So, ideally, this requires a connection to national labs. However, there is a very different way how the programs operate in the national lab environment and academic environment. I realized that I don’t know much about the real world because by virtue of being in academia, essentially, since high school, I have a very good idea about the scientific landscape but much less appreciation of how the real world works. I knew quite a lot of people at Google, Facebook, and Amazon. I tried to reach out to them and see where I could spend a year in the industry.
Q) Why limit your time to just one year?
SK) A lot of my colleagues on the theory side spend half a year or a year in places like Google or Facebook. So that seems like a good amount of time to both learn and enough to make a useful impact. Because more than a year is a change of career, less than a year is not enough time.
Q) What kind of lessons do you try to impart to your students before you know you send them off into the real world?
SK) One very important question is, what does it mean to become a PhD? A lot of my colleagues in the industry have the perception that having a master’s degree is enough to be successful in the industry. So why would you even consider the Phd degree? The answer is that, generally, you have two things: You have the skill set, and you have the opportunity to create something new. That’s what Phd means in the area of open research when you go out and do something new.
Interestingly enough, the industry will have equivalent types of positions in the engineering sense. For example, the role of the principal engineer is not to solve the problems that come from management; it is to find the new problems that management is not aware of and solve them before that. But the PhD means the opportunity to do the exploratory research independently on yourself. So you need to learn how to ask scientific questions. This is, in some sense, a criterion for graduation.
The second very important lesson is that, ultimately, the role of academia is to prepare people for the outside world, not to create a faculty for academia. So statistically, 95% of people graduate, if not 99%, go and work in industry. What is important is what you do in the real world and in terms of applications. I would be happy if one of my former students became a professor at places like MIT and Berkeley. But I would be much happier if they started a billion-dollar startup. For me, it would be a much stronger measure of the impact.
Q) How can people keep track of what you’re working on?
SK) I made the point of being visible on Linkedin at linkedin.com/in/sergei-kalinin-5bb44b18. I found it a be an exceptionally useful way to build relationships with people that I don’t know. I share my knowledge and experience. I use it a little bit as a combination of the blog and professional network. But as long as the gods of LinkedIn are happy about it, who am I to complain?